

Megaish is a free Windows app that allows you to create high quality vector images. Moreover, using the ViT -L/16 model, the performance can reach 56.7, which builds a new state-of-the-art for masked image modeling on ADE20K.Softonic review An easy-to-use and fun app for creating vector images.The above table shows that BEiT V2 significantly outperforms previous self-supervised methods.UPerNet task layer is used and the model is fine-tuned for 160K iterations with the input resolution 512×512.A longer pretraining schedule further boosts the performance to 87.3%. Meanwhile, BEiT V2 using ViT -L/16 with 300 epochs reaches 86.6% top-1 accuracy, which is comparable to data2vec with 1600 epochs.
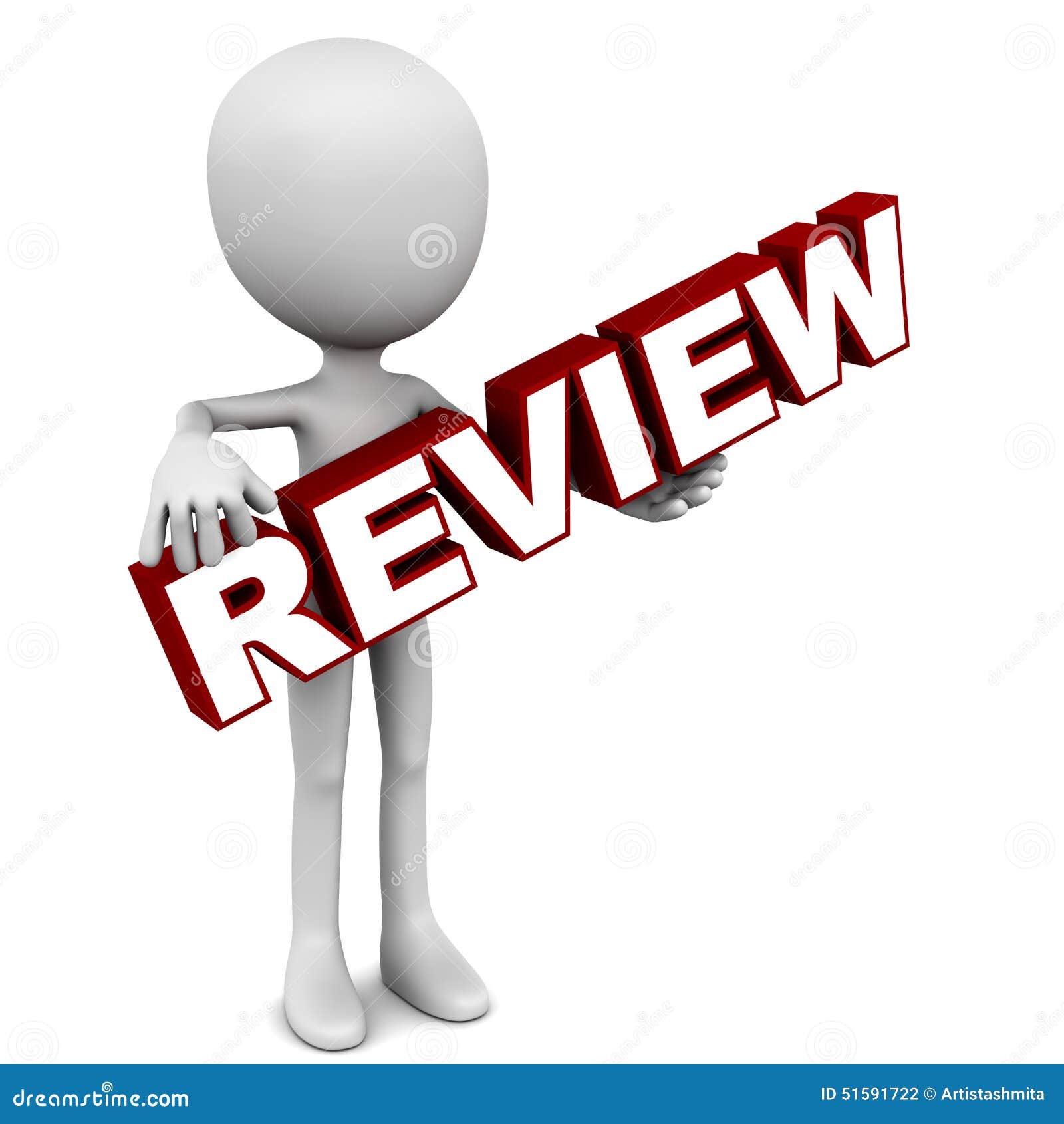
#IMAGE VECTORIZER REVIEW PATCH#
In order to pretrain the last layer’s CLS token hLCLS, it is concatenated with the intermediate l-th layer’s patch vectors.As illustrated in the above figure, a representation bottleneck is constructed to guide the CLS token to gather information.The goal is to mitigate the discrepancy between patch-level pretraining and image-level representation aggregation. The CLS token is explicitly pretrained for global representation.where zimeans the visual tokens of the original image, and D represents the pretraining images.
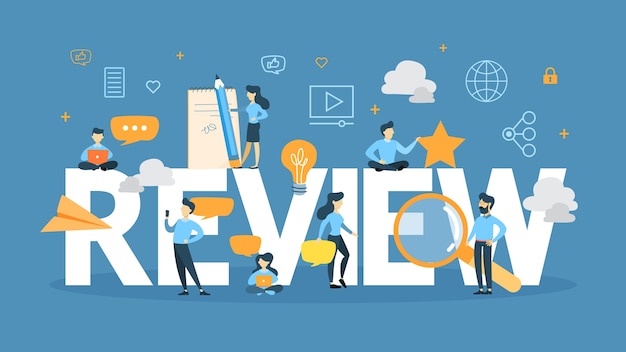
#IMAGE VECTORIZER REVIEW CODE#
For the i-th image patch, its quantized code is obtained by: Next, the vector quantizer looks up the nearest neighbor in the codebook for each patch representation hi. The tokenizer first encodes the input image to vectors. The tokenizer is consist of a ViT encoder, and a quantizer.VQ-KD has two modules during training, i.e., visual tokenizer, and decoder.Vector-quantized knowledge distillation (VQ-KD) is proposed to train the visual tokenizer.To be specific, the image x is tokenized to z= ∈ V, where the vocabulary V (i.e., visual codebook) contains | V| discrete codes. The visual tokenizer maps an image to a sequence of discrete tokens.Then the image patches are flattened and linearly projected into input embeddings for Transformers.ViT is used as backbone, which splits each 224×224 image into a 14×14 grid of image patches, where each patch is 16×16.Training process of visual tokenizer, which maps an image to discrete visual tokens 1.1.
